The US Army has awarded a Phase I Small Business Innovation Research (SBIR) funding to Autonomous Solutions Inc (ASI) to develop a deep learning (DL) architecture to support sensor fusion in GPS-denied environments.
Funding was awarded by the US Army Combat Capabilities Development Command Ground Vehicles Systems Center.
ASI chief technology officer Jeff Ferrin said: “Environmental sensing today typically includes cameras, LiDAR and radar. Each of these devices has a specific purpose, but not all of them work well in every situation.
“For example, cameras are great at collecting high-resolution colour information, but do not provide much useful information in the dark.”
Poorly lit or degraded visual conditions pose challenges to not only cameras, but also LiDAR and radar sensors.
The performance of LiDAR can be affected in fog, heavy rain, snow or dust, due to its use of light spectrum wavelengths.
How well do you really know your competitors?
Access the most comprehensive Company Profiles on the market, powered by GlobalData. Save hours of research. Gain competitive edge.
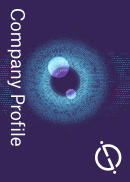
Thank you!
Your download email will arrive shortly
Not ready to buy yet? Download a free sample
We are confident about the unique quality of our Company Profiles. However, we want you to make the most beneficial decision for your business, so we offer a free sample that you can download by submitting the below form
By GlobalDataRadar is designed to penetrate such environments, but ‘often lacks spatial resolution’, ASI said.
Ferrin added: “ASI’s goal is to design a deep learning architecture that fuses information from LiDAR, radar and cameras. We plan to build upon machine learning techniques we have already developed for LiDAR data.”
Deep learning is a type of machine learning and artificial intelligence that allows users to extract relevant information from vast amounts of data.
The grant funding will allow ASI to address the gap in existing deep learning research efforts for LiDAR and radar.
The army intends to have improved data utilisation for a better understanding of a vehicle’s surroundings.
In the grant solicitation, the army stated: “It is anticipated that harnessing a wide variety of sensors altogether will benefit the autonomous vehicles by providing a more general and robust self-driving system, especially for navigating in different types of challenging weather, environments, road conditions and traffic.”
Under the Phase I SBIR contract, ASI will showcase the capabilities of the deep learning architecture in a simulation environment.